Subproject "Applications for Shared AI"
S3AI takes up and bundles requirements from the companies, reflects them from the point of view of integrity and privacy threat scenarios, comprising:
- Specification of requirements from the company partners’ application scenarios in terms of safety, information security and trust and elaboration of test scenarios for benchmark analysis;
- Benchmark analysis regarding privacy and robustness against various threat scenarios and against standard deep learning models;
- Guidelines for industrial partners as basis for R&D strategy development regarding the S3AI objectives;
Objective, Milestones and Target Values
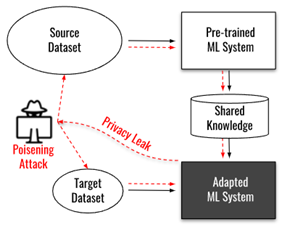
S3AI addresses performance and security challenges of shared AI, also referred to as collaborative AI or federated learning between different data sources and models, as illustrated by the shown figure. In this context we encounter the risks of i) performance degradation by data shift and ii) security threats. The most relevant security issues result from adversarial poisoning data attacks to corrupt the system or inference attacks to spy privacy-critical data.
This application scenario occurs in a variety of application scenarios when limited data prevents restriction to a single data source. For example, when an ML model is deployed for a customer, it needs to be tailored to the actual data of the application, which is usually different from the data at training time in a lab environment. The retraining at the customer site raises transfer learning and security challenges. The transfer learning challenge is fundamental by calling for an essential extension of the classical setting of statistical learning theory. The need for lifting the model under consideration with the help of data or derived artifacts from a different source raises particular security threats to the integrity of the system. The main threats come in terms of adversarial attacks of data poisoning or membership and inference attacks. While the first category of threats refers to the intended behavior of the system, the latter addresses confidentiality and privacy issues with respect to the source data.